Projects of the Data Research, Access and Governance Network (DRAGoN)
An overview of our current and completed research projects. In addition, we also provide regular training and consultancy.
Current projects
Qualidata Use and Confidential Knowledge (QUACK)
- Project lead: Elizabeth Green
- Project team: Professor Felix Ritchie
- Partner organisations: ICPSR, University of Michigan, GESIS, University of Mannheim, Heidelberg University, UK Data Archive
- Funding: UWE Bristol internal funding
- Expected completion date: December 2022
When research data consists of personal identifiable information, there is a risk that publishing analyses will inadvertently release confidential information about data subjects. Quantitative data has well-established practices to manage this risk. As well as statistical theory, there are widely used practical guidelines and teaching materials. Research in statistical disclosure control (SDC) was mostly sponsored by national statistics institutes (NSIs), which provided both the motivation and the market for the research.
In contrast, there are almost no guidelines for qualitative data. Researchers working with qualitative data must trust to their own judgment and experience, often without any training or mentoring. As well as increasing the risk of confidentiality breach, this is inefficient, as each generation must learn the same lessons for itself.
One reason for this is the sheer range of qualitative data: ethnographic studies, social media analyses, interviews, videos, clinical case studies, court records. Guidelines in one field may be meaningless in another. A subsidiary reason is that there is no equivalent of the NSI network to sponsor qualitative data research. The Qualidata Use and Confidential Knowledge (QUACK) project seeks to scope and develop both principles and guidelines for working with disclosive qualitative data.
EREOSDC (Outputs Statistical Disclosure Control)
- Project lead: Professor Felix Ritchie
- Project team: Dr Ben Derrick, Dr Laura Fogg-Rogers, Elizabeth Green, Dr Laura Hobbs, Professor Jim Smith, Professor Paul White
- Funding: UWE Bristol internal funding
- Expected completion date: December 2022
Data is everywhere. Increasingly the data used for policy and analysis is confidential and needs to be protected. Analytical uses of data are moving to ‘trusted research environments’ (TREs), where users have great freedom to analyse data, but what they produce gets checked before release into the open to minimise confidentiality risks. UWE Bristol is a leader in telling people how to do this, but we are currently fragmented and running to stand still. This project is designed to allow us to
- strengthen the theoretical foundations of what we do, including public engagement
- develop re-usable resources for use by us (and others, with appropriate recognition
- cement UWE Bristol’s reputation as a world leader in data governance
- integrate and develop IT solutions UWE Bristol staff have built as pilots
There are four strands to output checking:
- Checking of statistical outputs: UWE Bristol has the field almost to itself and is the leader
- Checking of qualitative research outputs: an almost completely unresearched field
- Checking of Artificial Intelligence (AI) outputs and machine learning models: a completely unresearched field
- Statistical Disclosure Control (SDC) for national statistics; a well-established and very competitive field
Guidelines and Resources for Artificial Intelligence Model Access from Trusted Research Environments (GRAIMatter)
- Project leads: Emily Jefferson (University of Dundee), Professor Jim Smith
- Project team: Professor Jim Smith, Professor Felix Ritchie, Dr Richard Preen, Dr Francesco Tava, Andrew McCarthy
- Partner organisations: University of Dundee, University of Edinburgh, Durham University and others
- Funding: DARE/Health Date Research UK
- Expected completion date: August 2022
Researchers from the College of Arts, Technology and Environment at UWE Bristol are part of a consortium awarded £390K by the UKRI as funding for GRAIMatter (Guidelines and Resources for Artificial Intelligence Model Access from Trusted Research Environments) under a scheme run by DARE-UK which is developing a national research data infrastructure for the UK.
This project will investigate what technical, legal and ethical frameworks would enable Trusted Research Environments to safely manage the risk to individual’s privacy when allowing the release of AI-based models learned from the confidential data they host, such as medical records. Such models could have significant value for the public good - for example, diagnosing disease risk.
Professor Jim Smith (Computer Science Research Centre) is leading on technical AI-related aspects with Professor Felix Ritchie providing expertise on procedural/researcher-focused aspects and Dr Francesco Tava (College of Health, Science and Society) on ethical issues.
This project ties into the interdisciplinary DRAGoN which involves a number of College of Arts, Technology and Environment researchers, and which was recently awarded 100K investment from UWE Bristol's Expanding Research Excellence strategy.
Wage and Employment Dynamics (WED) - Phases 2 and 3
- Project lead: Damian Whittard
- Project team: Arusha McKenzie, Van Phan, Professor Felix Ritchie
- Partner organisations: University College London (UCL), Bayes Business School, University of Reading; NIESR
- Funding: ESRC
- Expected completion dates: July 2023 (Phase 2) and March 2024 (Phase 3)
The Wage and Employment Dynamics (WED) project’s primary aim is to develop a sustainable, documented ‘wage and employment spine’ with the potential to fundamentally transform UK research and policy analysis across a vast range of topics. Alongside the creation of data infrastructure, the project will also generate research findings of direct interest to policy makers. Public benefit will be maximised through the provision of high-quality metadata and training for users.
Student Continuation
- Project lead: Professor Felix Ritchie
- Project team: Dave Allen, Dr Tim Hinks
- Funding: UWE Bristol internal funding
Very often, questions of EDI (equality, diversity, integration) are framed in overly simplistic ways: “What proportion of [ethnic group X] had first-class degrees?” and so on. This ignores the complex pathways that have led to those outcomes: who progresses at each stage of their career, and who drops out? Does it matter whether the student lives at home, or did a foundation year, or took a placement? What drives the choice of placement? Do some students to better at exams and some better at coursework? Does the gender/ethnicity/some other characteristic of the teachers matter?
The aim of the project is to analyse student records try to give a more rounded picture on where and how differences in progression and performance arise amongst students. The expectation is that will provide the quantitative base for a follow-up qualitative study, leading to specific policy recommendations to improve student performance and reduce differences.
DRAGoN PIAP (Public Involvement and Advisory Panel)
- Project leads: Dr Laura Fogg-Rogers, Dr Laura Hobbs, Dr Matt Studley
- Project team: Sam Graham, Elizabeth Green, Professor Felix Ritchie
- Funding: UWE Bristol internal funding
- Expected completion date: July 2024
Increasingly, academic research projects are being asked to demonstrate that they are engaging with the public. Public engagement can provide insights into ethics and the acceptability of projects, can help shape the direction of research, and can facilities dissemination and getting buy-in. This is most advanced in health research where public involvement and engagement (PIE) is often now a requirement for ethical approval for research.
However, these PIE panels are usually set up to cover specific projects. We are setting up a semi-permanent 'public involvement and advisory panel’ (DRAGON PIAP). The idea is to provide a long-term facility for UWE Bristol research staff to draw on where they want to engage with interested members of the public. This could involve helping researchers identify participants in community research projects, partners for funding bids involving local organisations, asking for public insight into research proposals at very early stages, perhaps even suggestions areas of research for UWE Bristol staff to look at.
This is an experimental project, and so this may go in different ways. However, we have committed to supporting it for two years initially, until July 2024. This gives us the space for DRAGoN PIAP to develop, perhaps in unexpected directions, but also to allow relationships to build organically without the pressure of a short project timeline. The first event will be a public engagement event in September 2022, organised jointly with UWE Bristol’s Turing Institute Network.
Completed projects
Wage and Employment Dynamics (WED) - Phase 1
- Project lead: Professor Felix Ritchie
- Project team: Arusha McKenzie, Van Phan, Damian Whittard
- Partner organisations: University College London (UCL), Bayes Business School, University of Reading; NIESR
- Funder: ESRC
- Project duration: October 2019-July 2022 (Phase 1)
Review of SDC options for output tables
- Project lead: Elizabeth Green
- Project team: Professor Felix Ritchie
- Funder: HESA
- Project duration: July 2021-March 2022
Externalities (wider costs and benefits) of data use (subcontractor)
- Project lead: Damian Whittard
- Project team: Professor Felix Ritchie
- Funder: DCMS
- Project duration: January 2021-March 2021
Process evaluation and R&D and innovation in data access and governance
- Project lead: Damian Whittard
- Project team: Dr Kyle Alves, Elizabeth Green, Professor Felix Ritchie
- Funder: Open Data Institute
- Project duration: October 2020-March 2021
Autumn school in data governance for low and middle-income countries
- Project lead: Professor Julie Mytton
- Project team: Elizabeth Green, Dr Francesco Tava
- Funder: NIHR
- Project duration: October 2020-December 2020
Advanced ethical models of data governance
- Project lead: Dr Francesco Tava
- Project team: Elizabeth Green
- Funder: UWE Bristol internal funding
- Project duration: September 2020-August 2021
Automated disclosure control for research outputs
- Project lead: Professor Felix Ritchie
- Project team: Elizabeth Green, Professor Jim Smith
- Funder: Eurostat
- Project duration: January 2020-December 2020
The value of data governance
- Project lead: Damian Whittard
- Project team: Professor Felix Ritchie
- Funder: CABI
- Project duration: July 2019-December 2020
You may also be interested in
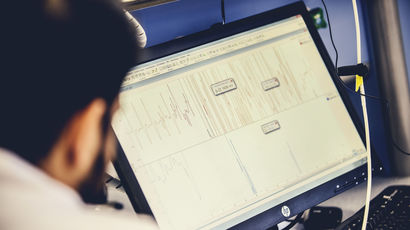
Risky business: Taking the fear out of data management
UWE Bristol research has led to changes in public data sharing laws around the world after proving that a risk-averse approach was ineffective.
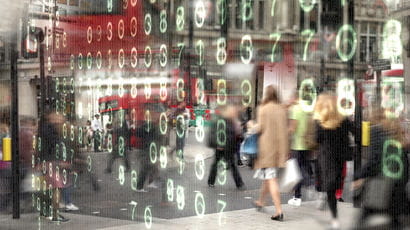
Safeguarding public data
Fresh insights into artificial intelligence, are transforming the way confidential data is processed and publicised by UK government and statistical bodies.